The development of new drugs is a complex and time-consuming process.
In the fast-paced world of medicine, the development of new drugs is a complex and time-consuming process. However, recent advancements in the integration of artificial intelligence (AI) have opened up new possibilities in the field of drug discovery. By leveraging AI technologies, researchers are revolutionizing the way we find new treatments for various diseases.
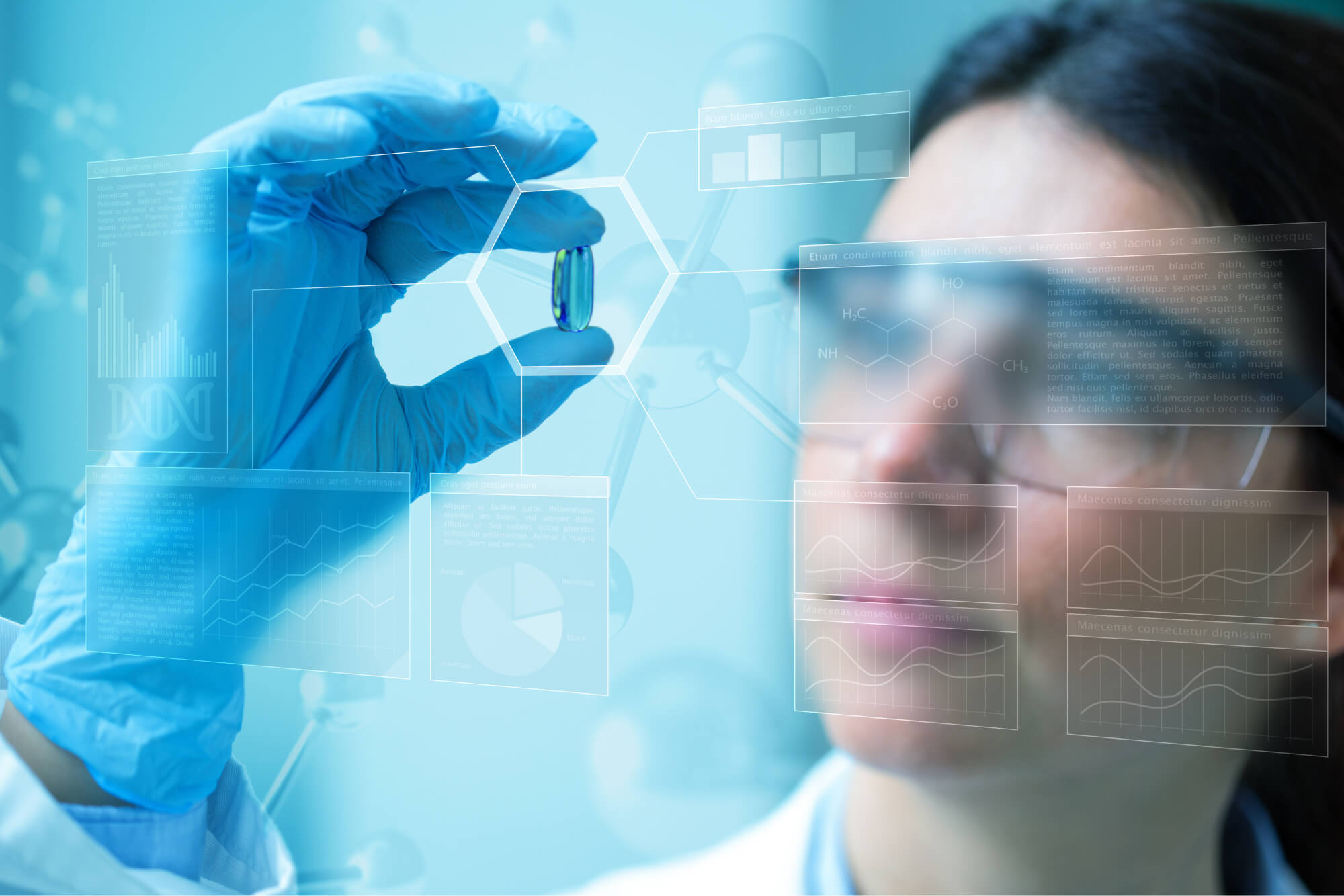
Drug repurposing, also known as drug repositioning or drug reprofiling, is the process of identifying new therapeutic uses for existing drugs and is the first area of your drug development pipeline that AI and ML can help streamline. By leveraging large-scale data from various sources, including drug databases, clinical records, genomics, proteomics, and scientific literature, AI/ML algorithms can analyze and identify potential drug candidates for repurposing. These algorithms can uncover hidden relationships, identify shared molecular targets or pathways, and predict the likelihood of success in new indications. By streamlining the process of identifying and evaluating drug repurposing opportunities, AI/ML accelerates the development timeline, reduces costs, and increases the likelihood of success in bringing new treatments to patients more quickly and efficiently.
Another of the critical challenges in drug development that BIOiSIM helps with is understanding drug-drug interactions. When multiple drugs are administered simultaneously, their interactions can have significant implications for efficacy and safety. By incorporating AI algorithms, researchers can analyze vast databases and predict potential interactions between drugs. This enables them to design better drug combinations and optimize treatment regimens for patients, minimizing adverse effects and maximizing therapeutic outcomes.
A third crucial aspect of drug development that can be assisted by AI/ML is drug formulation. AI algorithms can assist in optimizing drug formulation by analyzing various factors such as chemical properties, pharmacokinetics, and patient-specific characteristics. This includes predicting solubility and permeability of drug compounds, ensuring optimal delivery and bioavailability. These predictions can enable researchers to tailor drug formulations to enhance efficacy, stability (shelf life) and improve patient compliance.
Patient stratification is another area where AI is making a profound impact. Rather than employing a one-size-fits-all approach, researchers can identify patient subgroups that are more likely to respond to a particular drug. By integrating patient data, such as genetic profiles and clinical characteristics, with AI algorithms, researchers can predict treatment response and select patients who are more likely to benefit from a given therapy. This approach not only improves treatment outcomes but also reduces unnecessary exposure to potentially ineffective drugs.
In clinical trials, artificial intelligence is revolutionizing the way efficacy predictions and safety-toxicity profiles are generated as well. By utilizing large datasets from clinical trials and real-world evidence, AI algorithms can extract valuable insights, identify patterns, and make accurate predictions regarding a drug's effectiveness and safety profile. This allows researchers to make informed decisions, optimize trial design, and increase the chances of success in bringing new treatments to market.
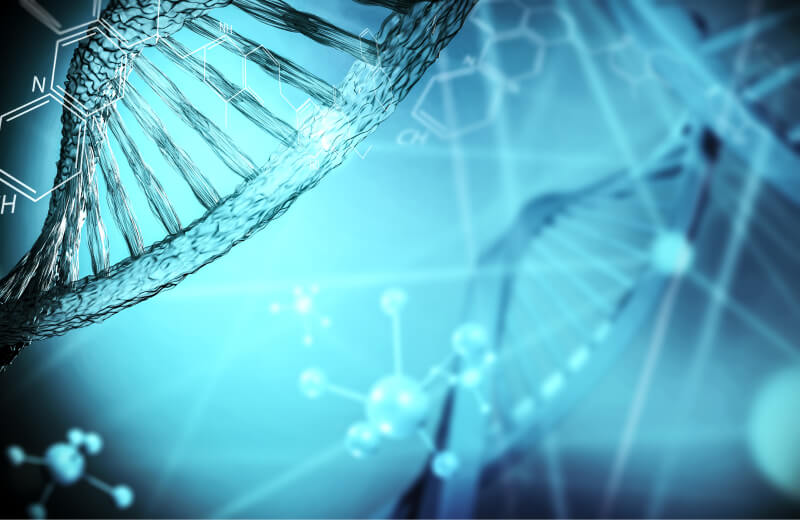

Biosimulation, another powerful tool in drug development, combines mathematical modeling and computer simulations to simulate biological processes. By creating mechanistic models that capture the complexity of the human body, our engineers created a platform that can predict drug responses and simulate clinical trial outcomes. Biosimulation aids in bridging the translational gap between preclinical and clinical stages, providing valuable insights into drug efficacy, safety, and dosing strategies.
AI can also be a drug development game-changer in the context of dose optimization, ensuring that patients receive the most effective and safe treatment regimens. By leveraging vast amounts of data, including preclinical and clinical trial data, real-world evidence, and patient-specific information, AI algorithms like those used in BIOiSIM can analyze complex relationships and patterns to determine the optimal dosage for a particular drug. These algorithms consider various factors such as drug pharmacokinetics, pharmacodynamics, patient demographics, genetic profiles, and co-morbidities. The models can then identify dose-response relationships, predict drug concentrations in different tissues, and assess the impact of factors like drug-drug interactions or patient variability on dosing requirements. By providing personalized dosing recommendations, AI helps reduce the risk of under- or over-dosing, ensuring that patients receive the right amount of medication for their specific condition. This optimization process ultimately leads to improved treatment outcomes, enhanced therapeutic efficacy, and minimized risks of adverse events, thus advancing the field of drug development and patient care.
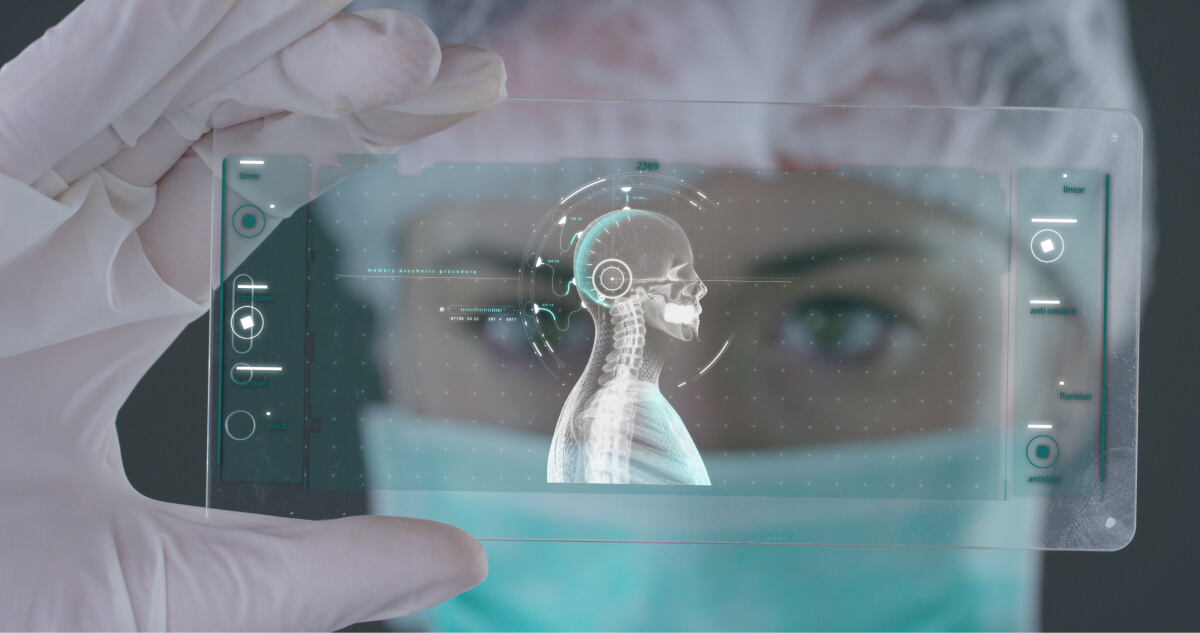
Generative AI and de novo discovery are two more powerful tools reshaping the landscape of drug development. Generative AI algorithms can create novel compounds by learning from vast chemical and biological databases. By generating new molecules with desirable properties, generative AI opens up new avenues for identifying potential drug candidates. This approach accelerates the drug discovery process by quickly exploring a vast chemical space and potentially uncovering molecules with unique therapeutic properties.
On the other hand, de novo discovery focuses on starting from scratch, without relying on existing drugs or compounds. It involves the design and synthesis of entirely new molecules based on specific target characteristics. By leveraging computational modeling, virtual screening, and AI algorithms, de novo discovery enables researchers to explore uncharted territories and discover novel compounds that may have significant therapeutic potential. Both generative AI and de novo discovery offer exciting opportunities for finding innovative drugs and pushing the boundaries of traditional drug development approaches.
AI technologies are also playing a vital role in animal reduction. By employing gene expression profiling and molecular modeling techniques, researchers can gain valuable insights into the mechanisms of drug action without relying solely on animal experiments. This not only reduces ethical concerns but also accelerates the drug development process.
In the realm of pharmacokinetic modeling, AI is enhancing our ability to predict drug chemical absorption, distribution, metabolism, and excretion (ADME) in the human body. By analyzing large datasets and integrating various factors such as chemical properties, protein characterization, toxicity, efficacy and patient-specific information, AI models can generate more accurate pharmacokinetic models. This allows researchers to predict and prioritize drug candidates with favorable absorption profiles early in the development stage, reducing the need for extensive and costly experimental testing. These predictions help optimize drug dosing, minimizing side effects, and maximizing therapeutic efficacy. They can incorporate patient-specific factors such as genetics and demographics to enable personalized medicine approaches, tailoring drug formulations and dosing regimens to individual patients.
Lastly, biomarker discovery is a critical aspect of personalized medicine and targeted therapies. AI algorithms can sift through vast amounts of genomic and proteomic data to identify potential biomarkers associated with disease progression, treatment response, or adverse events. These biomarkers can guide researchers in patient stratification, predicting treatment outcomes, and developing companion diagnostics.